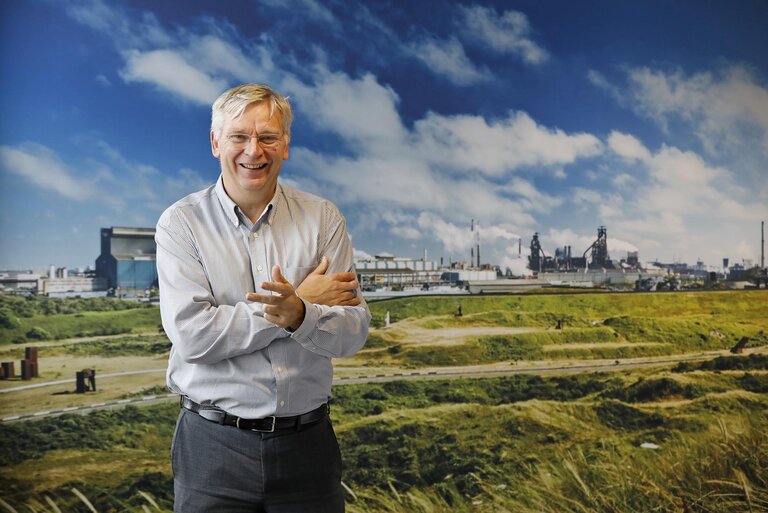
Tata Steel working on DataDrivenSteel
Effective use of data can greatly improve your business processes. At Tata Steel, they know all about it. Menno van der Winden, General Manager Advanced Analytics, shares some best practices and talks about how they are working on it at the giant industrial complex in North Holland.
In his own words, Menno van der Winden is a "hardcore metallurgist" who became gripped by big data and data science. After studying at TU Delft and obtaining his PhD at the University of Sheffield, he ended up at the then Royal Hoogovens, later Corus, and now Tata Steel. 'My fascination with the possibilities with data began in 2016 with research into a persistent quality problem in one of our 16,000 products’, says Menno. 'We couldn't get to the root cause of it until we deployed data and machine learning to find out where it went wrong. Then I suddenly experienced the added value of such tools. From that time on I went further and further with Artificial Intelligence (AI) and machine learning, which led to more use of data to improve our processes.'
AI is stupid
In this deployment of data, Menno has sought very limited help from outside consultants. 'We have a lot of substantive expertise here about our materials and processes. And for me, the added value is mainly in the combination of materials expertise and an AI application. Because in itself artificial intelligence is stupid; it only becomes intelligent when experts add valuable and correct data to it. Otherwise you create nonsense.'
Four priorities
In recent years, Tata Steel launched the term "data-driven steel." Menno: ‘By this we basically mean the digitization of the entire business process. In this, we work with four priorities. The first is AI and machine learning: how do you make decisions based on data streams? The second is dashboarding. This is super important and ensures that the knowledge gained is visualized. Compare it to a modern car: it's so packed with technology that it can make all kinds of decisions on its own, and yet it has a dashboard where you as a driver can see all the relevant information yourself and you can make decisions based on that. As a third priority, we have different apps. Those are little programs that facilitate repetitive processes. That can range from robotic process automation to a QR code to order specific bolts. And fourth and last is about "data across the chain." We want to start integrating as much of our data as possible with that of suppliers and customers. Ideally, that data-sharing process runs from the mine where our raw materials come from to the recycling plant where the finished products are prepared for reuse. Preconditions for this are security - we want to maximize data security, of course - and data governance, because the quality of the tools depends on the data used.'
Goals
And what do you achieve with all that data use? 'Deployment of data has several goals for us,' says Menno. 'We use it for sustainability targets: less energy consumption, reduction of CO2 emissions, prevention of odor nuisance and dust. There are also economic targets, for example gains in efficiency, better quality and less use of energy and raw materials. Moreover, we use it to relieve our employees. After all, there is already a great shortage of technical workers, so the more we can automate the better.'
Just get started!
Other industrial companies can also benefit from data, is Menno's firm belief. And the threshold for doing so need not be high at all. 'My most important advice is: just start! Think about what your biggest challenges are, for example in your maintenance, your quality or your production speed. These are typically topics in which you can make improvements with data. Then you can start engaging your employees. Look around your organization. Are there perhaps people you already know who have an interest in data? Perhaps you know someone who has already made their entire house "smart"? Or someone who scours the Internet for how neural networks work? Chances are such a person would be happy to participate in an internal training or a specific data project. Many organizations have a lot more data talent in-house than they think. And there is also quite a lot of information about smart data use online that you can start with yourself.'
Many organizations have much more data talent in-house than they think.
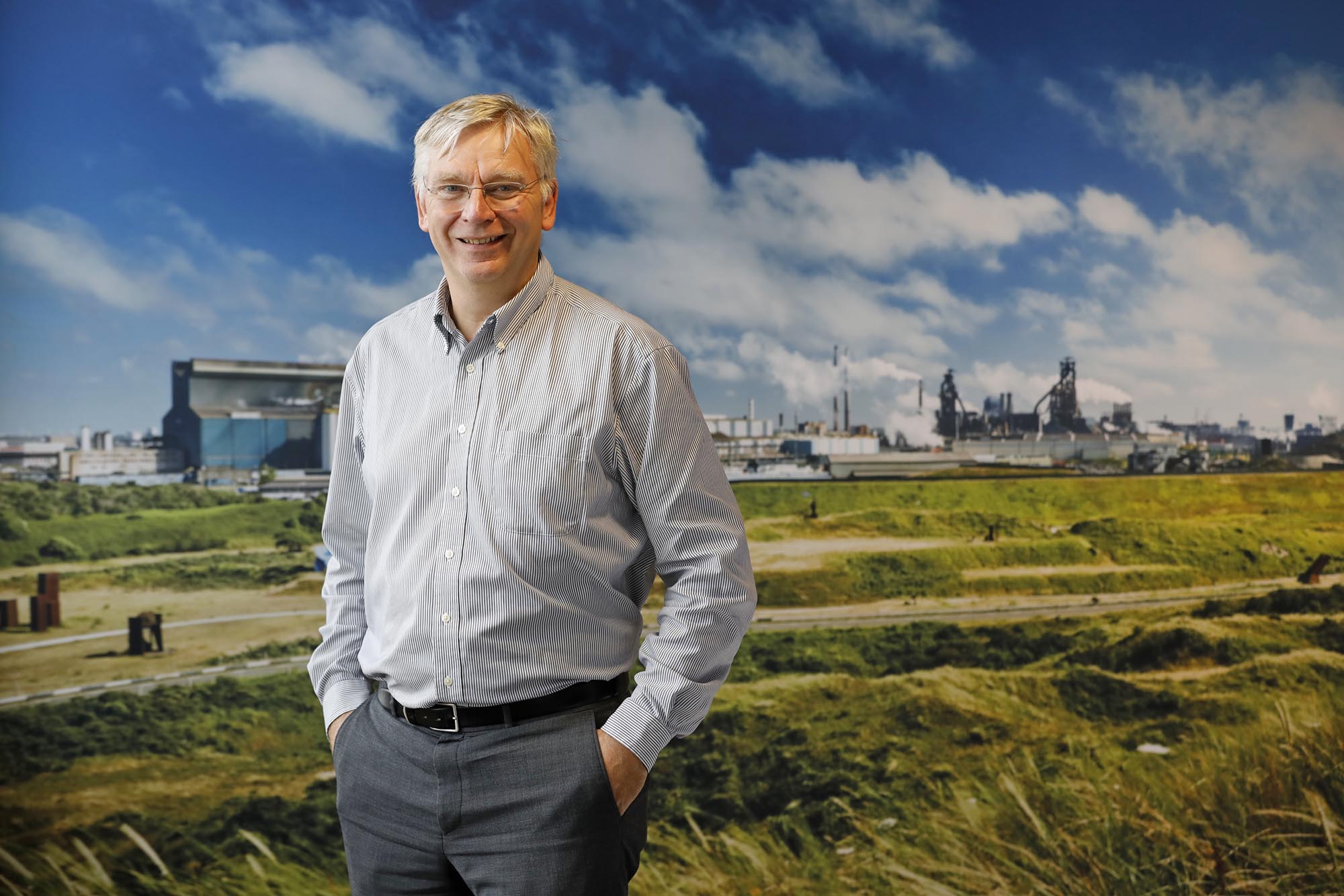
Menno van der Winden
General Manager Advanced Analytics
Pitfalls
Of course, there are also pitfalls when you start with data science. 'It must not become hobbyism,' warns Menno. 'Because of course we have experienced that too. That's why we agreed that every data project must have a "use case" that describes "who" can do "what" with the system in question: your project must solve a problem or we won't start it. In addition, you have to understand very well that sometimes people are not waiting for your genius solution at all. So you also have to think about how to "sell" your solution and bring about a truly sustainable change. Data analysis and the resulting possible improvements are virtually limitless, but it's all about applying them in practice. How do you ensure that the knowledge gained is correctly applied even at 3 a.m. by the operator in the control room? That is now a much more important question.'